NimbleEdge utilizes cutting edge technology to enable
Hyper- Personalization
NimbleEdge integrates edge computing, federated learning (FL) and differential privacy with its cloud-based PaaS to offer companies an end-to-end solution for hyper-personalization driven by real-time ML.
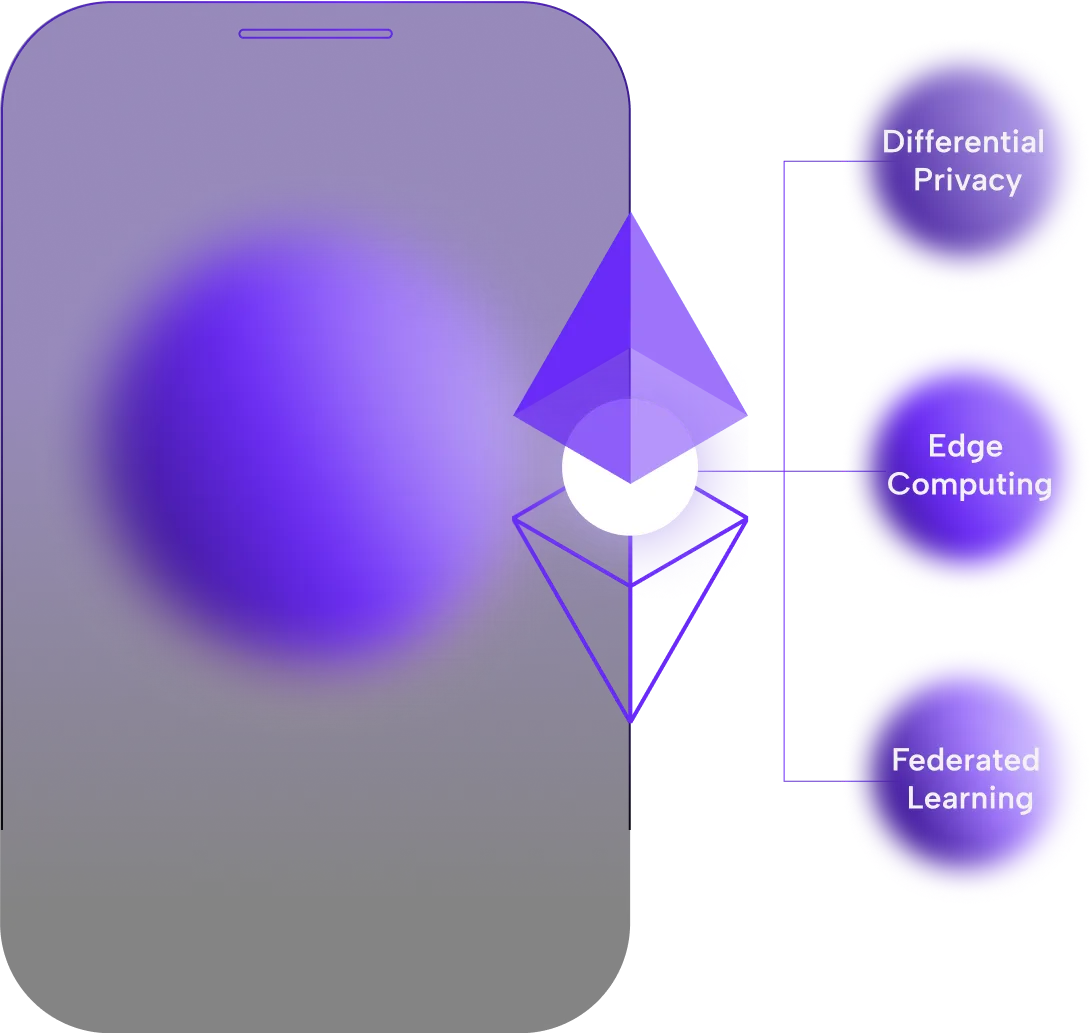
Take advantage of the compute power of edge devices like smartphones
NimbleEdge’s technology swings the pendulum back from the centralization of compute in the cloud to take advantage of the compute power of edge devices like smartphones. Before NimbleEdge, executing real-time AI/L pipelines required complex, data intensive processing and network intensive communications resulting in exorbitant cloud costs.
Edge Computing
Edge computing is a computational architecture that locates data processing and storage on edge devices like today’s smartphones.
There is a constant upgrade in capabilities of edge devices i.e. smartphones. Yet, many apps still rely on the cloud application architectures rather than leveraging the computational power of the phone.
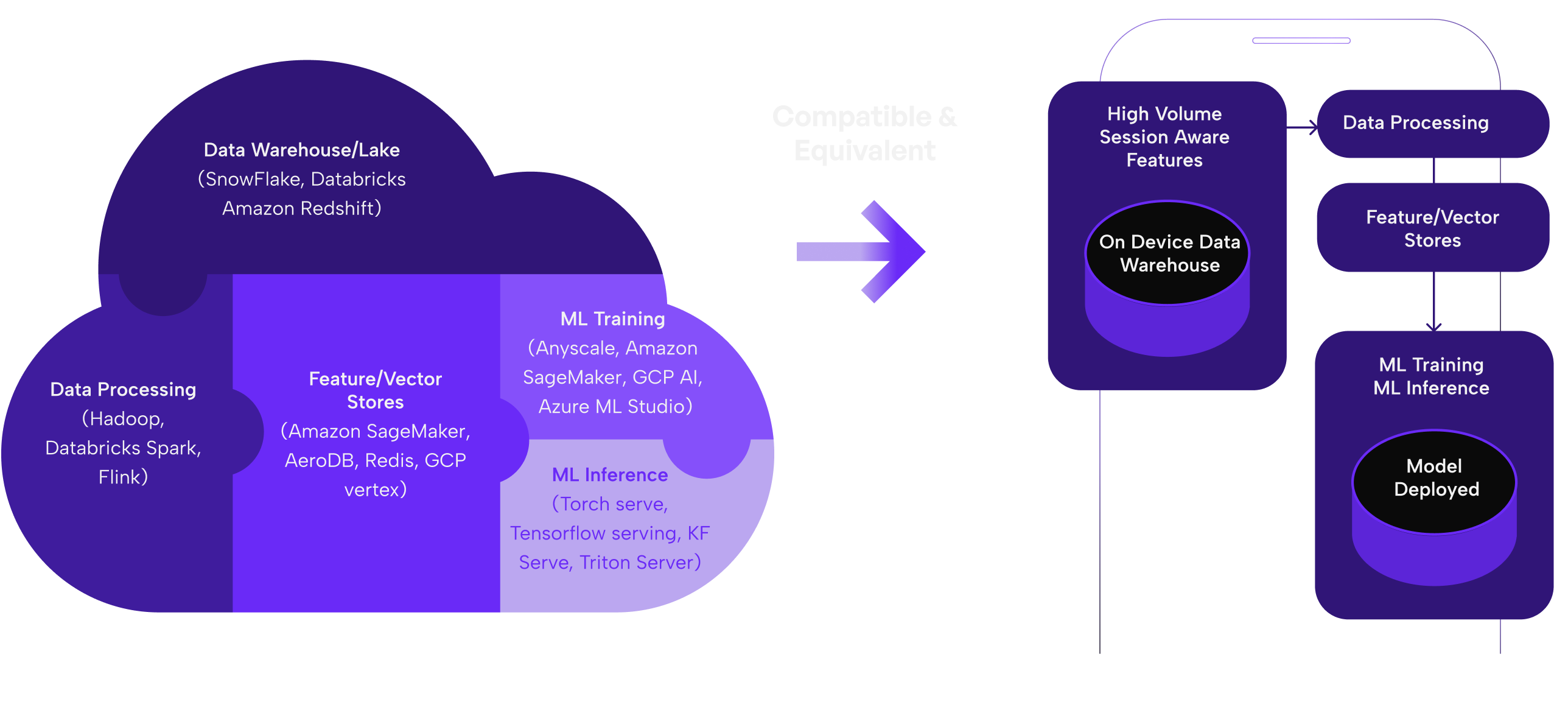

~1000x Faster
Today’s smartphones are almost a thousand times faster than the mid-’80s Cray-2 Supercomputer, several multiple times faster than the computer onboard NASA’s Perseverance Rover currently exploring Mars and - perhaps most significantly - faster than the laptops most of us are carrying around today.
Operational complexity is simplified by intelligently balancing the ML workloads
Distributing the workload of data handling to the device enables real-time hyper-personalized ML engagement because the ML pipelines are executing locally. Latency is reduced to human imperceptible levels. Network activity is minimized. User privacy is protected. PII is not transmitted to the cloud. Operational complexity is simplified by intelligently balancing the ML workloads - inference and training - between the NimbleEdge cloud service and the edge device.
Federated Learning
Federated Learning (FL), also called collaborative learning, is an innovative ML technique that enables the training of AI models on user devices without requiring the user device to share its local data. Essentially, each user has their own personally trained model - a million models for a million users - that uses a vast pool of heterogeneous data available at the edge.
Federated Learning offers better generalization patterns across different models resulting in more relevant results.
The NimbleEdge service aggregates the locally trained models and excludes any PII data from being transmitted. The central server combines local models to create an updated global model that is sent back to the edge, enabling machine learning without sharing data while ensuring privacy. Federated Learning offers better generalization patterns across different models resulting in more relevant results.
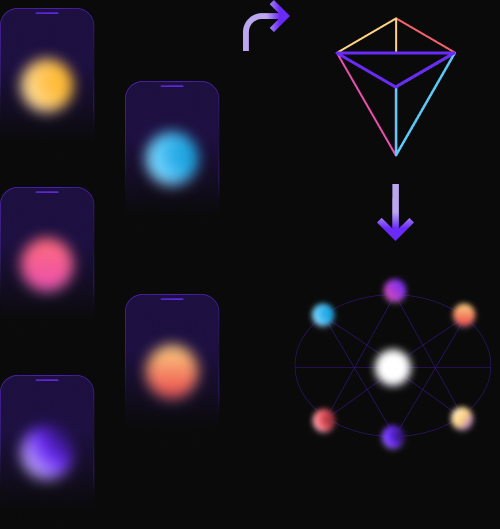
Differential Privacy
Differential privacy is a rigorous mathematical definition of privacy. An algorithm is differentially private if from its output, you cannot discern if an individual’s data was or was not part of the original dataset.
By adding “random noise” to a dataset, a differentially private algorithm can publicly share information about the dataset by describing patterns of groups in the dataset while withholding information about individuals.
No compromise on individual privacy with Differential Privacy.
NimbleEdge has implemented differential privacy to help organizations develop ML-based recommendation models that collect and share aggregate data while maintaining individual privacy.
Leverage the Intelligent Edge for Your Industry
NimbleEdge Platform manages both orchestration and execution capabilities for on-device ML
on-the-fly updates
Orchestration
Platform
Want to
learn more?
