Getting Started with On-device ML in E-commerce: Use Cases and Benefits
.png)
Introduction: Understanding the Consumer
Steve Jobs said, “People don’t know what they want until you show it to them.” It is true that many solutions to people’s needs are entrepreneurial feats of imagination that must be demonstrated in a tangible way for consumers to recognize the value. However, this wisdom is also applicable in more subtle ways.
For instance, consumers often are not conscious of the many points of friction that they encounter during shopping experiences on native mobile applications. Yet, E-commerce companies can cater to “what people want” in ways that they may never consciously realize by “showing” the user down individually custom-tailored paths that reduce or remove these points of friction. The data subsequently affirms through decreased abandon rates and higher average order values that consumer “wants” were met.
Using technology to capture and interpret behavioral and contextual signals allows companies to show people personalized solutions that multiply the impact of surprising and delighting consumers.
Advancements in machine learning (ML)-driven personalization, in particular, allow organizations to take Steve Jobs’s wise words and apply them at incredible scale. Mckinsey’s Future of Personalization report quotes that machine learning (ML)-based product recommendation systems could lead to a 5-15% increase in revenue and 10-30% efficiency in marketing spend. E-Commerce companies have begun exploring these avenues to capitalize on these opportunities.
Hyper Personalization at Scale using ML
Personalization is nothing new to E-Commerce. E-Commerce companies who have implemented personalization strategies have seen remarkable performance uplift from doing so: Those with faster growth rates than their slower-growing counterparts derive 40 percent more of their revenue from personalization, according to McKinsey. These results have motivated organizations to explore ML as a means optimizing their personalization implementations.
However, companies grapple with the challenge of personalizing experiences for millions of daily users, each with complex interaction patterns. To manage this data volume, e-commerce needs robust data science pipelines for large-scale and advanced levels of personalization. While cloud-based near real-time ML solves several limitations of batch ML systems, it also creates dependency upon cloud infrastructure and its associated costs. In addition, cloud-based ML is subject to issues such as traffic spikes, delayed response times, limited personalization options, and increased risks to user data privacy.
These performance challenges may appear insurmountable, while the entire endeavor itself may also seem completely cost-prohibitive.
How can E-Commerce companies achieve real-time ML-driven hyper-personalization while keeping costs contained and avoiding performance problems?
The Answer Lies at the Edge
Organizations can realize the previously untapped potential of utilizing edge devices to deliver truly real-time ML-driven personalization. NimbleEdge has created a solution that captures real-time user interest and delivers one-to-one personalization to the user in a cost-efficient way at scale through our on-device managed ecosystem that replaces the ML cloud stack. By processing data directly on the device, it minimizes the need to transmit large amounts of data to the cloud, thereby reducing latency, saving cloud processing cost, and enhancing data privacy.
The E-commerce industry, in particular, can realize 3 key benefits from this on-device machine learning approach:
- Search Recommendation
Based on Mckinsey’s value of personalization report, 76% of customers expect personalized experiences in online shopping. NimbleEdge enables session-awareness through the comprehensive capture of a user’s interactions in a single online session, paired with real-time engagement features that empower the platform to instantly offer tailored search recommendations in reaction to user behavior. These hyper-personalized search recommendations not only delight customers, but also benefit the retailer by yielding increases in add-to-cart rates and average order value (AOV).
Watch the video below to see how NimbleEdge helps your E-commerce app be session-aware and present relevant recommendations to your audience based on real-time capture of their activity.
- Homepage Item Discovery
Providing quick item discovery functionality in your app prevents consumers from haphazardly browsing content that doesn’t align with their interests, leaving them fatigued and disenchanted with their shopping experience on your platform. NimbleEdge leverages session engagement data to generate homepage recommendations in real-time for expedited item discovery that reduces choice paralysis and product search time.
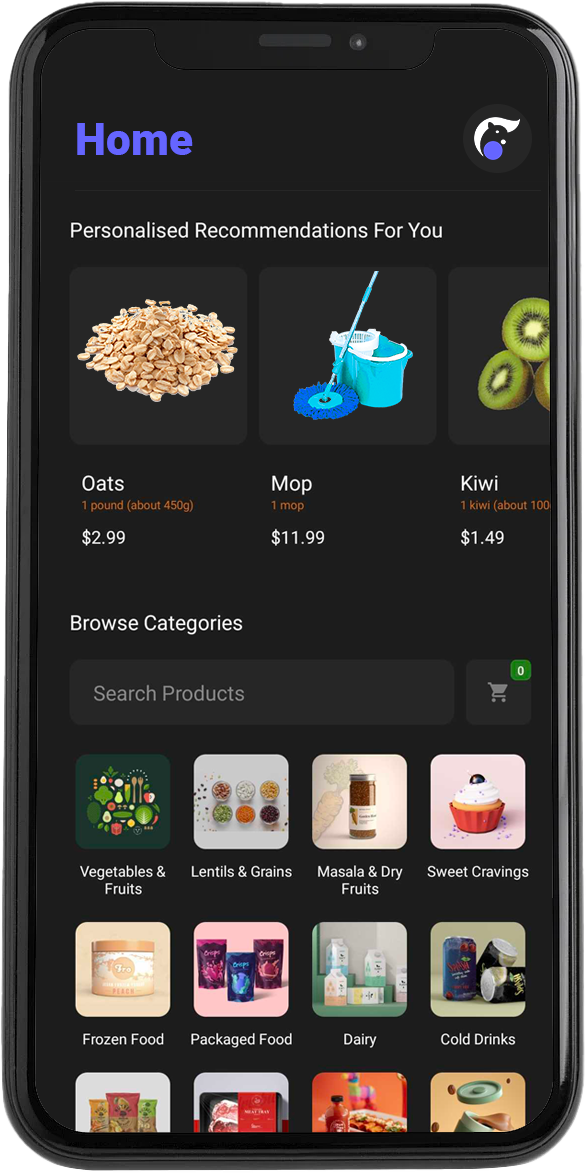
- Checkout Cross-sell
When a customer reaches checkout, their cart contents and session activity data can powerfully inform the decisions concerning what cross-sell offers to dynamically display as they move through the final steps to transact. NimbleEdge equips E-commerce apps with the real-time processing capabilities to instantly present more relevant cross-sells with higher conversion rates, increasing AOV by up to 9% and reducing cart abandonment rates due to reduced latency.
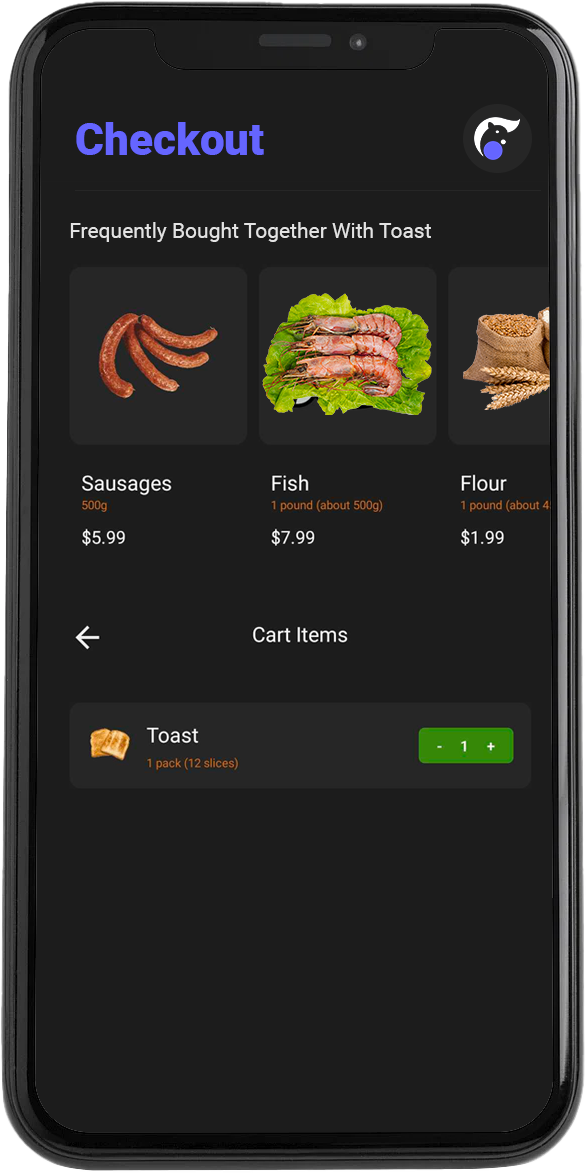
Summary
A personalized digital shopping experience today is no longer a nice-to-have but a necessity for company survival in the impending AI age, as customers defect to competitors who are excelling in how they harness personalization.
NimbleEdge allows E-Commerce companies to improve and scale ML models’ performance while reducing the operational complexity and associated cloud costs. If you would like to learn more, get in touch with our team today.
Read more on how we helped an e-commerce customer
- Save 50-60% in cloud costs
- Achieve 6% uplift in ML model performance
- Smoothly handle 7x scale-handling during through-put burst
- Go live in less than 2 weeks time-to-market
{{cta}}